2024 IT Priorities and Adoption of Gen AI
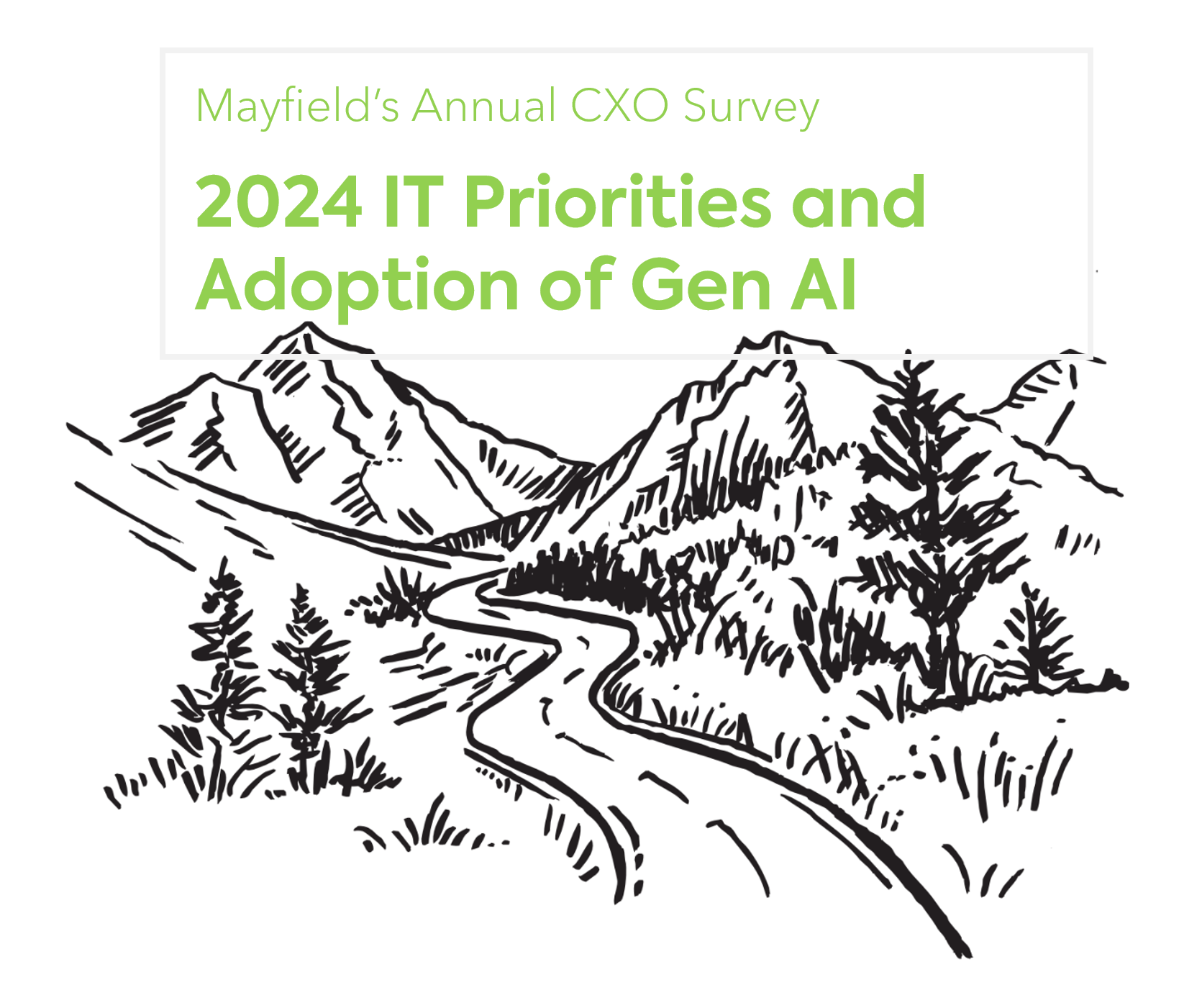
Today we welcome Todd James, Chief Data & Technology Officer at 84.51º, to The CXO Journey to the AI Future podcast. He joined 84.51º in August 2021, where he paved the way for continued growth, building on its rich data, science, and technology capabilities. Previously, Todd spent 15 years at Fidelity Investments where he held a variety of key strategic leadership roles. As an innovative leader, Todd built the global data and analytics organization for Fidelity’s Workplace Investing and Health Care business units. He also led efforts to modernize servicing and operations through applied artificial intelligence and automation and directed Fidelity’s Cross-Enterprise AI Center of Excellence (COE).
Question 1: First Job: Could you talk a little bit about your background and how you got to the position where you are now?
We’re a retail data science organization that is part of The Kroger Company. As I like to think about it, what we do is we help Kroger run a media operation that goes to market.
We also have an insights business that helps CPGs and partners understand the path to purchase, and a venture capital fund called PearlRock Partners that invests in emerging food services businesses. Additionally, we’re the advanced analytics arm of the parent company of Kroger. So when it comes to AI and advanced analytics across Kroger, we’re tasked with driving a lot of those results across the organization.
It’s interesting that throughout my career I’ve moved between business leadership roles and technology leadership roles. So while I was at Fidelity, I held a variety of responsibilities from running business units to strategizing the organization. The tail end of my tenure there was really around building out data and advanced analytics capabilities for the workplace, investing in healthcare businesses, and setting up the global capabilities for that. So I had a great opportunity working at Fidelity to do a lot of different things, including help build out the advanced analytics and AI journey that they’re on today.
Question 2: Generative AI: Everybody’s talking about it. You’ve spent a lot of time with data and analytics for years. Could you put a little context around Generative AI? How is the Gen AI movement different?
It’s different, but a lot of the capabilities aren’t necessarily new. There are things happening today that we’ve already been doing for 5-7 years. The difference is that what was very difficult to do (and on a limited scale from a usage POV), has become a lot easier to implement. It’s far more democratized.
The capabilities are greatly expanded relative to some of the natural language solutions that we were working with several years ago. As I look at it, I think the democratization of AI is what has been unlocked. We put incredible power, in terms of prediction, into the hands of more people. And I think that will open up all kinds of opportunities to simplify and enhance customer experiences, making the lives of our associates easier. So I do think there’s a bit of a shift, and a lot of it has to do with accessibility.
Question 3: What are some of the early learnings in Generative AI for you?
First of all, if you talk to most people, I think the rate of change we’ve become accustomed to continues to compress. What we’ve seen over the last year, and even what we’ve seen with some of the recent announcements over the past couple of weeks, is that the acceleration of capabilities and the acceleration of benefits that you can get from this technology, is unprecedented.
So how you think about your business, how you think about managing it, and how you think about up leveling it has put a lot of pressure on existing structures. I think from my perspective, our goal has been to solve how you shift from a world in which the majority of your analytics are developed, supported, and maintained within an analytics organization, to one in which the analytics organization is part of a much bigger ecosystem in which businesses are using out-of-the-box capabilities with AI, third-party vendors are coming in, and the capability is being deployed far more broadly. So that’s a bit of a learning curve.
The good news is, if you have solid processes in place, and you have solid controls, then you have a basis around which you can start to build things out. I would imagine that if you don’t, you’re probably feeling rather exposed right now.
Today, everywhere we have a decision point being executed either by a person, through automation, or via AI (through prediction), we always talk about how to have the right control process and the right control framework. If it’s in a manufacturing facility you may have in-line quality checks. If it’s technology, we have deployment checks and monitoring around that. It’s no different, I think, with AI. You need to embed your solutions within a control framework. And that was probably a little bit easier to manage when everything was coming out of an analytics organization. Now you have to think about that at a different scale. So that’s definitely one of the learnings.
Another big one for us was engaging the business on the journey. To some extent, the exhilaration and excitement in the media around Generative AI has grabbed a lot of attention. And it’s grabbed a lot of attention, not just for Generative AI, but for how advanced analytics and data can have an impact and transform the business in general. So there’s been a real opportunity to have more discussions around the organization that enables us to work on some of the change aspects and educational awareness with business leaders.
The third learning is that you have to scale. It’s very hard to generate value through individual point solutions. But…if you build a capability in a way that’s beneficial on one decision point, and then another decision point, and another decision point…you can do that at scale. And that’s where you start to see some of the opportunities.
Some of the solutions out there are inherently built to scale, but I think a lot of the use cases basically amount to: solve this problem here, solve that problem there. But what you come to realize is that these solutions are just text summarization around a particular set of information that could potentially be used by a much broader range of employees. Ideally, you want to make that available so you can tailor it to individual populations. That’s where you can start to see some of the real benefits.
Bonus: This concept of ‘Buy versus Build’ is often a technology decision that you, as an IT leader, have to make. And with Generative AI, it feels like we’re at the beginning of a whole new set of technologies. What’s the ‘buy versus build’ thesis that you believe we should be thinking about?
I think it’s a little bit of a forcing function because of the speed and pace in the space today. The underlying fundamentals on this haven’t really changed. We should be building things in-house that are truly proprietary and differentiate us on a competitive level. That’s where I would prefer to put our assets and our professionals who are focused on building data and analytics products.
However, what I’m seeing is that the platforms are accelerating a lot of the capabilities that they’re offering from a Generative AI point of view, especially around commoditized capabilities, and we want to be able to leverage where they’ve already put in the work. So we’re additionally having discussions with our partners about what’s on their roadmap. For example, there have been a few cases where we saw tremendous value in leveraging Generative AI to better equip people to answer questions. However, after talking to our partners, we realized that was already on their roadmap. So we’ll apply our resources in other areas for the time being.
The third consideration around “buy vs. build” is that there are certain cases where we see unique emergent capabilities that we don’t currently possess the skill to advance. For example, startups that sit outside the large platform providers. Many startups have very unique capabilities on the outside that can accelerate a niche area where we want to drive value. So we’re working with emerging technologies as well.
I think because of the pace at which everything is moving, some things we thought were pretty novel ideas, we realized, were cases that just about every company wants. So we need to figure out just how much of the world is already on the large providers’ roadmaps. This enables us to assign our resources to other tasks that help us become more competitive.
Question 4: What are some of the issues, or maybe even gaps, in technology that you believe need to be addressed? I know this isn’t a technology-only issue. What are the headwinds that you have to address?
You have to think about scale to really understand the gaps. Point solutions are easy. You can go online and say: “Give me a recipe for a rustic Italian meal that is inspired by this part of the Italian coast.” Anyone can do that. It makes it seem very easy. It sets everyone’s expectations super high, before they start to see the gaps.
The real challenge is when you want to evolve it at scale in a way that can support your business. First, the tech stack starts to evolve. We went from a traditional tech stack that we had become fairly accustomed to, to a data and analytics tech stack. Now, we have to figure out how we want to incorporate it into both market-based and proprietary LLMs, and additionally fit everything into our architecture and the supporting set of capabilities around it. A lot is still being defined, it’s very new. The majority of companies, unless you’re actually a tech services provider building these capabilities, probably have a gap now because it’s novel.
For the other gaps, I think we need to take a hard look at changing the way we work. One of the discussions that we’ve been having with the business is: How are these capabilities going to change how we work? What are the skills and competencies that we need to start building within our organization? How do we help our people on that journey? Where are we incorporating new tools? Where are we working with our teams to grow?
You have to shepherd people along the path. Not because people don’t have the capability, but because it’s a chain. You need coding, but you also need prompt engineering. To some extent we now have solutions that we’ve deployed inside the business, and we’re working with people that are not technologists. So we have to ask: How do you leverage some of the Gen AI capabilities that we provided? How do you write prompts? How do you write good prompts? How do you evolve? How do you get the right information out? So initially it’s going to be about raising everyone’s skills and knowledge on the business side.
The final gap is going to be ensuring that this technology, like any manual or technical process that you’re building out, has the right, and most efficient, controls.
At the end of the day, the mindset needs to be less “I’m going to build an individual chair as a craftsman” and more “I need to build a factory that can produce furniture at scale.” The same thing is true here. I don’t need a use case. I need a system that can support multiple use cases in a way that is efficient, effective, reliable, and responsible.
Question 5: Responsible AI: How do you think about it? What does it mean to you?
It means a few different things. One of which I would say is that no matter what industry you’re in, you’re in the business of trust. You need to make sure that you’re thinking about responsibility and you’re thinking about how you’re using this technology in the right way.
Additionally, we want an environment where we’re bringing in and hiring people who can feel confident about the processes we’re going to exhibit and sustain, and the ethics around how we’re doing work. So we’ve put a lot of time and effort into refreshing some of the practices that we had around responsible AI. This includes enabling them to be ported across the broader organization, which brings us back to that concept of democratization. We have a framework in place that’s pretty focused on making sure that our AI is reliable and performative. It does what it’s supposed to. It’s compliant with privacy and trust. It’s secure. But also: It’s safe. We’re in the business of food. We want to ensure that our algorithms are performing in a way that accounts for the safety of our associates, our customers, and society at large. There needs to be accountability as we deploy these solutions, which goes along with transparency and explainability.
Finally, the other key aspect that we take a hard look at is around fairness. At the end of the day, our goal at 84 51º is to make people’s lives easier.
I’m very fortunate to be in an organization where we want to have a positive impact on the world. We want to make sure that what we’re doing from an analytics perspective reflects all those values, and all those ideals, both inside the company, but also with regards to the people we attract to come work here, who have similar standards and similar expectations.
Todd James is currently the Chief Data & Technology Officer at 84.51°, a retail data science, insights, and media company helping The Kroger Co., consumer packaged goods companies, agencies, publishers, and affiliated partners create more personalized and valuable experiences for shoppers across the path to purchase.
A driver of digital transformation, Todd spent 15 years at Fidelity Investments where he held a variety of key strategic leadership roles. An innovative leader, he built the global data and analytics organization for Fidelity’s Workplace Investing and Health Care business units. He also led efforts to modernize servicing and operations through applied artificial intelligence, and automation and directed Fidelity’s Cross-Enterprise AI Center of Excellence (COE).
Prior to Fidelity, Todd led a strategy consulting practice at Deloitte, directing strategic engagements with global Fortune 500 and government clients. As a director at Resource Consultants, Inc. he built and led a technology services business unit while also overseeing corporate IT. Prior to his business career, Todd was an officer in the U.S. Coast Guard where he held leadership roles in IT, information security, and shipboard operations.
Aside from a B.S. in Mathematics and Computer Science from the U.S. Coast Guard Academy, Todd also earned an MBA from The College of William and Mary, and a M.S. in Computer Science from the University of Illinois. He is an editorial board member for CDO Magazine.
Today we welcome Sathish Muthukrishan, Chief Information, Data, and Digital Officer at Ally to “The CXO Journey to the AI Future” podcast. He joined Ally Financial in January 2020 with a focus on modernizing processes, teams, and technology and driving transformative business outcomes across multiple Fortune 500s.
Question 1: First Job: Could you talk a little bit about your background and how you got to the position where you are now?
Today I serve as Chief Information, Data, and Digital Officer, and it’s a really huge privilege to be serving under that title at a digitally native bank. Because fundamentally, everything we do centers around technology. It’s a dream job for any IT leader who wants the accountability and responsibility to make everyone else around them look extremely successful.
I originally grew up in the airline world, starting with Singapore Airlines and then United. I later moved onto other verticals: American Express and Honeywell before joining Ally Financial.
Question 2: Generative AI: Everybody’s talking about it. Undoubtedly you have been doing AI analytics and other things in and around automation for years. Could you put a little context around Generative AI? Is this the highest priority for you right now?
I may have a slightly different philosophy and take, but I’ll get to your question. I’ve believed for a long time that we should never lead with technology. If you lead with technology, you take your eye off what’s important, which are the capabilities you’re building for your customers. The technology must be relevant to the customer, and must drive business impact.
So we’ve always led with asking whether or not this is the right capability for the customers. Is it creating the right business impact? And then you figure out what technology you have to use to build that.
However, your question is very important right now, because sometimes a technology will come around that can disrupt every industry and every company out there globally. Gen AI may accomplish exactly that. So, there’s an extreme focus on how we can securely (and scalably) utilize gen AI, while still keeping in mind our two core principles:
Question 3: New Metrics: There are lots of use cases where Generative AI could be used. How will you go about deciding where to put attention? How do you go about defining the right metrics for an AI deployment? Is it productivity? Is it business impact? What are those metrics that matter?
Everybody is thrilled and excited to start using gen AI as you can imagine, and we have taken a very simplistic approach: Anything that we do with gen AI should either drive efficiency or drive effectiveness. Efficiency means an additive technology to make your everyday job better, easier, or more productive. Effectiveness is driving real business impact and results.
So how do we go about that? We know that this isn’t a technology that can be executed within IT – the entire company will have to participate, and it will have to become a revolution. So we created what we call an Ally AI Playbook. The AI playbook provides a platform for anybody who has an idea to take that idea to production. This could mean finding a path, figuring out the governance, learning who the right people to engage with are, getting access to the tooling, and moving everything forward.
In order to bring this playbook to life, we’ve created a step-by-step process:
That’s how we’re approaching our AI use cases.
Bonus: Everyone has been moving towards SaaS and no one is building their own custom solutions anymore. Do you believe that to be true? What’s the ‘buy versus build’ thesis with regards to AI that we should be thinking about right now?
Oh, what a great question to ask! And the right one to ask right now.
My take is that it’s buy and build, not buy versus build. Now I’ll tell you why. There are several reasons:
The first one is if you go buy generative AI and use it, you’re going to produce the same outcome as every other company. So how are you going to differentiate yourself?
And that leads to number two: If you go and buy off-the-shelf software or any SaaS offering for that matter, they’re using AI. And if you don’t play with it yourself and understand how it works, how are you going to leverage the AI capability that someone else is providing?
Finally, cybercriminals have the same access to this technology that we have. They’re trying to use it for proactive measures. They’re trying to use it to get a compromise from any company. So how do you then protect against that?
For all of these reasons, if your organization does not fully understand the impact that AI can provide, and if your technology team does not have a hands-on understanding of the technology, you will be left far behind.
And that’s why we say: buy and build. The way it has come to life at Ally is that we quickly understood that this technology is moving way too fast and new competitors are coming every day, so we created what we call an Ally AI Platform. You can think of it as a bridge between the internal Ally and all its applications, and the external AI capabilities. It gives me access to go interact with a model, let’s say, OpenAI, which is what we’re connected with today. If I don’t like it, or if I want an additional model to compare it to, I can go and connect with a LLaMA model or a Bard, and we’re doing that in our lower environments. But in our production environments, we are just connected to OpenAI. So the Ally AI platform gives me the ability to point to multiple models and either choose the best option or get all the outputs and compare (or even combine them) and make it more relevant to Ally.
Question 4: What are some of the issues, or maybe even gaps in the technology, that you believe need to be addressed? I know this isn’t a technology-only issue.
There are different buckets and each of them has their own weight in terms of how strong these headwinds are.
The one that I particularly worry about is third-party risk. We have an existing footprint in terms of using third-party technologies. Do we feel comfortable? Have they secured their tech environment the way we would secure ours given the advancement in security threats?
And like I said, gen AI has democratized cyber attacks. The same cyber attack that’s going after the financial industry can go to the healthcare industry. So, how do we protect ourselves?
The second is the sophistication of these cyber threats. It’s become emotional. And it’s turning into parallel attacks, where you have to be on your toes and keep educating your entire organization all the time. That’s the second headwind that I think about.
If I look at it internally, what do I have to do? First is education. You can’t leave the organization behind. So you have to be patient in educating everyone and showcasing the success that gen AI brings. Show them that this is not rocket science. Everybody can use it. English is the most popular language in gen AI, so everyone can play a part.
I come from a regulatory organization. The regulators control partners’ risk compliance and legal audit and help them understand what we’re implementing and proactively identify risks that come along with it. This way they can twist their brains to figure out what other risks there are.
We need to push the organization forward, but if we’re upfront about all the risks that we’ve identified, they can help us mitigate those risks. So that’s another headwind in terms of educating and bringing the organization along.
Then finally, there’s talent. How can you keep your talent challenged and give them better opportunities? You want them to stay with your company and not leave to join someone more sophisticated.
Question 5: Responsible AI: How do you think about it? What does it mean to you?
As a regulated institution, I have to think, breathe, speak, and eat Responsible AI.
One, because we need to have consistency of service. Our goal is to be a fair and responsible bank that mitigates model drift and bias, and this all starts with data cleanliness and accessibility. Further, we focus on testing our models ourselves, as well as the outcomes across various customer segments – to ensure that bias is not introduced.
Then, our independent risk organization tests the models out. For the first use case that we launched, they looked at 60,000 outcomes to make sure that there wasn’t model drift or bias introduced.
That’s from a business perspective.
From a social perspective, I worry even more. In this decade and this era, you have a lower chance of succeeding if you are not digitally connected.
You and I probably don’t think twice before we pick up our smartphones to connect and do what we do every day. But millions of people don’t have access to the internet, they don’t have access to a smartphone, or don’t have the education or opportunity to learn how to efficiently use them.
Gen AI, if not appropriately executed, implemented, and integrated within society, is going to widen the digital divide. And chances are it will become a digital abyss.
So, tech leaders are responsible for modernizing their organization with gen AI because they understand it the best. They can connect the dots. The public-private organizations have to make sure that they pay it forward to create a society that doesn’t become more divided on account of these digital advancements.
Sathish is currently the Chief Information, Data, and Digital Officer at Ally, a leading digital financial services company. Reporting to the CEO and a member of the company’s Executive Committee, he is responsible for leading product, user experience, data, digital, technology, security, network, and operations – an end-to-end role in the midst of everything that happens in the digital native organization.
Most recently, he was Honeywell Aerospace’s first CDIO with a charter to digitize the Aerospace businesses. As an example, he successfully transformed the used serviceable airline parts into a digital business powered by blockchain. He was also responsible for leading and transforming Honeywell AERO’s 10,000-plus global engineering organization.
Sathish was one of the pioneers of American Express’ digital transformation. He led the launch of several unique, industry-first, groundbreaking digital products enabling strategic partnerships with Foursquare, Facebook, Twitter, Microsoft, Apple, Samsung, and TripAdvisor steering the American Express journey from payments to commerce. He delivered the ability for Card Members to Pay with Points creating a new currency for payments.
Sathish’s experience in several technology leadership positions with Honeywell, American Express, United & Singapore Airlines has led to over 30+ patents in the manufacturing, payments, and digital technology space.
The Mayfield AI Pathfinders had their inaugural meeting this week on the Stanford campus, with the goal of building a thought leadership community for peer learning on key issues, trends, and innovations across the quickly emerging AI landscape. We think it’s important that investors and other thought leaders help bridge the gap between academics, entrepreneurs, and the actual enterprise practitioners and buyers, and see this as a great opportunity to cut through some of the noise.
Back when AI first got started, a lot of people didn’t believe in deep learning. So there was this phase of trying to convince everyone it was useful. Then we hit peak hype: AI can solve everything! – and that was the new normal. But we’ll come down from that to a place where AI is just a tool in the enterprise toolbox.
Computer science has been marked by several points throughout its history where people were researching a problem and came up with an algorithmic paradigm that didn’t work. So, researchers brute forced things, which led to an initial burst of innovation that ultimately tapped out. And in the end, innovators will always try to find a vector upon which they can scale and enable new capabilities. So, you’ll see these waves of algorithmic innovation that go through an S-Curve and tap out, become a tool, and then later, another thing is built on top of that.
Neural networks were on the rise in the early 90s. Support vector machines killed that, and almost all neural network research stopped around that time (from 1996-2006). But if we look today, in 2023, the vast majority of machine learning is still leveraging neural networks. That context is important because in 2006 the boom basically ended, but in 2023 we’re still seeing it.
So what about the next 10 years? Things are going to get tapped out, and something else will supplant it, build upon it, and do more.
As large organizations begin to explore using AI for some of their IT and business needs, there continues to be tremendous latent demand for enterprise software serving AI and LLMs today. However, there are still significant issues around access to compute, and these constraints are here to stay for at least the next 4-5 years. Moore’s Law is beginning to slow down and these costs are going to continue rising. This will be further exacerbated by geopolitical tensions with China and others. Unfortunately, this could be a limitation that enables only the most well-funded startups and incumbents to get ahead.
However, there’s a whole world of opportunity beyond scaling. To date, we’ve invested in scaling LLMs by using the dumbest most brute force methods. The dimensions of innovation still need to diversify, and much more is still needed at the design and architectural level. And this doesn’t just mean special-purpose models, it could mean models where the huge inefficiencies start to get ironed out, or working on things that aren’t models at all. Building a bigger transformer (so to speak) is only one way to skin this cat.
Furthermore, there’s still a huge need for specific solutions that serve end applications. It’s great that LLMs are so general purpose, which has added to their speed and propagation across the industry, but we don’t need or want that generality in 90% of the apps that will get used.
So, there will be still be a huge market for less costly pursuits, including:
For founders working on companies today, it’s important to think about whether or not you have a consolidated way to sell your widget across different verticals, because in that case you may have a good horizontal play. Look at the GTM action as your guiding force and try to be at the lowest point where the GTM action remains the same. Don’t go broad because then you’ll need dozens of different GTM motions, but don’t go too niche either, because then your market size is capped, and that’s basically the issue with every SaaS platform. Go to the lowest possible common denominator where the sales motion is the same or similar. It’s hard to have multiple GTM motions as a startup.
Additionally, it’s really important to be able to build a product at the right quality, cost, latency, metrics, etc. In the process of developing the product, it will often force you to focus and diminish its generality based on how early conversations with customers or deployments wind up going.
For large organizations, practical deployment of AI at scale is kind of the icing on the cake – the cake being your data, your infrastructure, your governance, your security, your privacy, etc. And if you don’t have everything, you can’t build an enterprise scale ML platform. You’re going to need lineage and traceability once regulations come in. It will be difficult for a startup to do something like that, but at the app layer there will be tons and tons of interesting companies coming up with new ideas.
So, IT and product teams are starting to get down to brass tacks after a year of evaluation: What does it take to deploy gen AI at scale in the enterprise? Fundamentally, the same considerations that were in place in 2017 still apply today. Things like cost, data cleanliness, etc.
For example, there are some incredibly interesting use cases in pharma where you could have a scientist ask an LLM to summarize all the experiments in the last five years on X molecule, and find out what the most common side effects are. The issue with building this out always comes down to the data. It’s a mess and it’s not complete. Enterprises still haven’t solved the fundamentals – but now the appreciation for these fundamentals is getting featured front and center. People want a car, but it has to have wheels and brakes.
When AI first hit the scene, everyone was talking about models and their capabilities, but that conversation is already becoming obsolete. Today, you can’t go to an enterprise company and tell them: “Look how awesome my model is.” Last year everyone was talking about “How many parameters?” (you wanted more), but now, not a single customer asks about the size of your model. In fact, if you tell them your model has tons of parameters, they will shut the door on you.
The business side of the house just isn’t interested in the same things as the technologists. They want to talk about their business use case and get help, not hear about what’s under the hood. What will it cost to get this in the hands of all my customer service agents? And what will the ROI of that be? People are thinking about putting these into production, they want an easy POC, and to figure out the ROI/Total Cost of Ownership. There were lots of multi-million dollar deals signed with Open AI so that companies could get boards and CEOs off their backs, but when it comes time for renewal, how many of these will even get renewed (vs. a downsell or churn)? People care about TOC and when you’re thinking about a 5,000 person contact center and giving everyone a chatbot and RAG implementation that equation becomes really difficult.
In the long run, cost may push people towards open source solutions as some of the talent gaps get sorted out – companies will just need a little help using their proprietary data and getting it into open source models at a reasonable cost long term. Today, there’s a 3-4x drop in price to train a model of a given size per year, being driven by, primarily, software and clever algorithms. If the cost falls by 8x, you’ll have way more people start working on these problems.
You want to evaluate technology at a high level, and if it’s transformational, you want to be investing right now. You can think about it using these three axes:
With those three considerations in play, the future may be pretty optimistic.
Since models are fundamentally building blocks towards a problem that a company is trying to solve, it may be that it makes more sense for large organizations to wait for their SaaS providers to build their own models in many cases vs. building something from scratch and then trying to compete. It will definitely depend on the use case, but many use cases won’t need a company’s proprietary data to bring value.
SaaS providers are already hard at work embedding these systems as part of their solutions offerings, many have gen AI departments and lots of smart people, but others are just trying to get an SLM to embed in an OEM model.
Aside from the obvious two issues: the costs around the underlying silicon systems, and shortage of valuable talent, there are a number of challenges companies are facing today when it comes to gen AI adoption.
In some cases, it’s hitting the cost-latency-performance profile, in others, it’s just not accurate enough. In regulated industries you may need more evaluation or explainability. So it depends on the particular use case or segment, different things will need to be solved for.
And on the business side, companies want to go fast, but they often have trouble defining the problem they actually want to solve. They need to pick one or two high value, high impact, problems and then properly define success. Today everything is just maximum hype, people want to do this shiny new thing, but they don’t know what it’s really capable of. Evaluation is important, but so is defining the problem you’re solving.
Additionally, it’s not yet clear how companies are going to take these models and successfully deploy them. No one knows what the killer apps are yet. It’s so new that everyone’s excited about it and it’s hard to disambiguate the hype and excitement from real market traction. People will pay for things right now, even huge deals, but it’s not sustainable yet. Where is the real traction vs. the hype?
Still, there are many silver linings, innovation in software and architecture will continue to drive down costs, but in some respects, the argument could be made that the hardest time has already passed. It’s a bit of a chicken and egg problem, if you don’t have enough capital you can’t scale up, but if you don’t show results, you won’t get the capital. Thanks to GPT, business users got a chance to see the hope/potential, so many incentives now exist to drive down costs by optimizing everything. Today, people are in a hurry, so they’re trying to quickly scale up, but in 2-3 years, as enterprises start to scale their solutions, there will be giant incentives to drive costs down. This is where the real innovation is going to start happening.
Furthermore, there’s a lot of capital going into this and it’s likely going to support larger and larger clusters. Public markets are trillions of dollars when compared with venture capital and IT budgets.
Training can only get so far ahead of inference, they’re interlocked. So, the more mature the industry becomes, the more people will start to recognize the role of inference. One of the big architectural innovations will be seeing how the relationship between the cloud and the edge evolves over time. We have constraints at the physics level that will put us in a place where the cost per useful unit of inference at the edge can be much lower than doing it in the cloud. So there’s a bit of a system architecture imperative to figure out what can be moved to the edge. When you’re talking about media AI, it’s a lot, maybe almost all of it. As we refactor the whole system to understand how inference can be redistributed in a way that’s appropriate to the end application, we’ll see orders of magnitude benefits around cost. It will become incredibly cheap to do some sorts of things at the edge, and will even influence what we choose to do. There’s an opportunity here besides just paying for high-end GPUs.
People’s time-to-solution is improving, which has a direct correlation with productivity, so everyone is motivated to build a more efficient datacenter. A >10x improvement could be achieved in memory if people just decided to focus on it. So much time has been spent in computing, but the movement time of data is also a huge setback. Today there’s finally market motivation to do something about it.
Additionally liquid cooling and other solutions are gaining popularity to help reduce electricity requirements – both for cost and sustainability purposes.
Deep learning is the most efficient way of solving large problems. It’s not winning for no reason and there isn’t an alternative that can solve the problem any cheaper today. Super large models will happen and need to happen. There are good reasons for them, just as there are good reasons for smaller models.
We can argue about OpenAI, but most would agree that it’s a very good model. Likely the best model out there today. In a steady state, there’s a real possibility that everything could go closed source with large vendors stealing the enterprise market. If proprietary models keep getting better and better, we may hit a point where it gets hard for open source to catch up. It may or may not happen, but the future market could very well be 80-20 (or more!) in favor of incumbents. Furthermore, when it comes to the most cutting-edge models, the dynamics of commoditization will likely take hold and prevent pricing from getting out of hand. However, at least for the time being, customers still want choice and will likely evaluate a number of different models.
Today, open source vs. closed source will ultimately depend on the goals of each individual organization.
First, open source will be much cheaper to scale, and that will create a counterweight against closed source models unless they pull ahead dramatically in performance. People are focused on getting apps up and running today, but later on they’re going to care a lot more about cost.
Second, it externalizes the cost of development and drives innovation much faster at scale.
Third, there’s way more flexibility inherent in open source. Companies can create domain-specific models, do whatever they want with the weights, and then run them on-prem or on-device. There’s a control aspect both around the business and around privacy. And additionally, there will be a huge role for a variety of deployments involving concerns around latency. There are a lot of industrial use cases today that are interested in using open source because people want to own the destiny of their product. You can think of it like in-sourcing vs. out-sourcing – there are pros and cons with each. If you outsource, you lose control of your destiny, IP creation, speed of updates, etc. People have data and that data can really become the differentiator. How you’ve cleaned that data is in itself IP. Customers will create their own custom filtering, which leads to certain model capabilities, and that model is then an expression of IP. Those incentives exist and will always lead to more models, whether that’s 100% rational or not.
Finally, open source is not just about being a good person and giving back to the community. There will always be a draw for smart people, because from a selfish standpoint, you can contribute something and take that longitudinally alongside your career. Your name and GitHub repo is on that project. Companies will then build products and services on top of that raw material.
There are great cases to be made about open source vs. proprietary. It comes down to what each enterprise wants. Most enterprises today don’t really want to train their own model from scratch. There’s a maturity curve at play here:
Companies start by experimenting with GPT-4, then prompt engineering an open-source model, then fine-tuning that model, then adding RAG, then pre-training, etc. Eventually, it’s possible to work your way towards building your own model.
But, it’s not a one and done: someone has to ultimately maintain these. Talent can leave and it can become really hard. You see problematic things like this all over the place in business today. For example, a team can’t move from excel into Google Sheets because someone wrote a macro 15 years ago and left. These sorts of problems will be further exacerbated if companies start building their own models.
It’s hard today to assess the safety of a system without good standards or a good way to audit. What if the data itself is biased, for example? As models proliferate, the harms are getting more and more specialized and teams all have different ideas on where to point the flashlight. A more profound consideration is whether you can even have safety at all without bias – everyone is making different choices and there are too many explicit value judgments. Regulation will be needed to help guide companies on what they need to do.
Today we welcome Balaji Viswanath, Managing Director of Emerging Tech, Enterprise Architecture, Software Engineering and RPA at Tyson Foods to The CXO Journey to the AI Future podcast. He joined Tyson in June 2018 as Senior Director of IT Architecture. Balaji is a Technology and Data Science executive with 25+ years of experience in his field.
Question 1: First Job: Could you talk a little bit about your background and how you got to the position where you are now?
I’ve had the privilege of being in the technology industry for about 26 years or so now. The first twenty of which I spent in a core technology company, and the last six in Tyson Foods. If I look back at my career, I’d say I spent the first third or so building technology, products, and solutions that we could use in-house. The latter portion was predominantly spent trying to deploy technology in a way that added business value.
If you look at core technology areas like e-commerce, digital marketing, big data, or very recently, Generative AI, you see over and over again that everyone is spending a ton of time in analytics. Everyone wants scalable analytics platforms that enable the business to gain actionable insights from their data.
Over the years it’s been a privilege to be part of high-performing teams. Recently, everyone is very passionate about what Gen AI can do for the industry and what Gen AI can do to add value at Tyson.
Question 2: Generative AI: Everybody’s talking about it. But you, as a business leader, have seen other cycles before. Could you put a little context around Generative AI? Is this the highest priority for you right now
I’m glad you asked that, Gamiel. From my experience and understanding, a very significant amount of prioritization has to be applied to AI today. During my day job, I speak to at least ten vendors a week or ten new product companies a week that are talking about AI in some shape or form. I do believe that the predictive components of what we do in our day-to-day jobs are starting to be omnipresent and very ubiquitous.
AI is different primarily because it’s evolving so rapidly. There’s a sense of “Oh, am I missing out?” So there’s an evolution, a paradigm shift. If you look back at the last year after the introduction of ChatGPT and its capabilities, I think the leap that Gen AI has taken as a technology has led to new products and new offerings every day. So, it’s starting to become a huge priority for most of the companies I speak to, including ours.
Extra question: You’ve been in technology for a while now. Could you talk about some early learnings? What lessons or even advice would you give to somebody who’s in an IT leadership position when it comes to taking advantage of Generative AI?
There are a few things I could talk about.
First: Start with the business problem. AI and data science, in an unprecedented manner, are forcing a convergence between what was traditionally IT and what was traditionally business. I think data science is bringing together these two disciplines very efficiently. So, start with the business problem and don’t go after tech for the sake of tech, or AI for the sake of AI.
The second thing I would say is to chew in “bite-size chunks.” One of the ways we try to explore Gen AI and try to bring it into our company is by starting with a small business problem. Engage very closely with your business partners, deploy something as quickly as you can, and see if you can get value out of what you deploy. And if you see value, then you expand on it a little more and make it big. But if you don’t see value quickly, it’s easier to fail fast and move on to the next thing.
And keep your options wide open. Today, every hyperscaler that we work with has a significant AI offering. It would be, in my opinion, short-sighted to think that we go with one and only one. I think all these offerings that we need are very nuanced in themselves, and I think having MLOps and foundational platforms that allow us to exploit the best capabilities from each and every one of these offerings would also be a key learning that I’ve gathered over the last few months.
Question 3: New Metrics: You and I talked a little bit about your way of measuring impact or metrics. There are lots of use cases where Generative AI could be used. How will you go about deciding where to put attention? How do you cut through all of the potential use cases with a metric that matters? And what are those metrics that matter?
That’s a very good but very complex question. This is where I probably have the greatest number of discussions in my day job. How do you determine the value of something once it’s deployed? Today, there are broadly two kinds of value that we look at.
One is the value that impacts the bottom line, which is around efficiencies and manufacturing…anything we can do to get more efficient as a company. And what AI and Gen AI can do to help us get more efficient.
And the other area of value that we look at is what we call “top-line value and growth.” Does this help us improve our top-line growth?
There’s also a significant amount of thought around how we can employ AI to improve our employee experience across the company.
I met a few people recently that were specifically focused on using AI to do opportunity sizing. So we can say, “Hey, even before you embark on the opportunity, what AI can we use to size this opportunity?”
So that’s our approach. We engage with our business partners and try to get as close to articulating value as possible (in terms of a dollar figure). We approach problems in small iterative chunks, fail fast, move on to the next thing, and hopefully, we’ll arrive at a good value proposition for a large AI project.
I will tell you, though, that an integral aspect of value is essentially the benefit minus the cost. So one of the things that we’re working on actively with all our partners is: “What does Generative AI really cost?”
We have still yet to meet a company that has truly figured this out. Because there’s a computing aspect to it. There’s a training aspect to it. There’s a big data aspect to it. There’s an analytics aspect to it. So we’re hyper-focused on trying to understand what Gen AI really costs and use that to understand its true value.
You’ve been in an industry that has been in the process of transforming its landscape to a cloud-based, SaaS model, and that mostly doesn’t build custom solutions anymore. Do you believe that to be true? What’s the ‘buy versus build’ thesis that you believe you should be thinking about?
Being in enterprise architecture, this is a question that we are confronted with very frequently. Do you buy? Do you build? We started off with a very traditional base layer type approach where we said: “Hey, if it’s a system of record and if somebody in the industry already does it extremely well, then why don’t we go buy it instead of trying to build it?” Versus anything we think is differentiated, we try and build.
When you talk about this traditional tenant, as far as AI is concerned, especially for Generative AI, it’s too early for us to see if we have to buy, or if we have to build. I do think there are use cases where it makes a lot of sense for us to build. I think there are two ways of looking at this. One is either we send our data out or we bring the AI in.
So, there are a few use cases where it makes sense for us to bring open source or other models inside the company, train them with our data, deploy, and see value.
There are a few other bigger use cases where we need the firepower of externally well-trained, hyper-scaler hosted, managed models. We typically build a strong decision tree around this.
Question 4: What are some of the issues, or maybe even gaps in technology that you believe need to be addressed?
Like I already mentioned, I think the first thing we’re trying to figure out is: What is the true cost of Generative AI? What is the true cost of deploying and scaling a Gen AI product inside a company like ours and seeing value? So that’s our first area we’re trying to understand.
And from a skills standpoint, prompt engineering is starting to become an extremely important skill. I did speak with a few people recently who were starting to invest in AI through utilizing prompt engineering which I thought was very, very interesting.
Prompt engineering is a skill that we’re starting to understand and is starting to be a multi-disciplinary thing. It’s not so much only a pure technology play. It’s not like you get the best programmer to write the best prompts. It’s starting to become a very interdisciplinary thing.
And last, but not the least, compute. We have been in discussions with a few people who are willing to deploy AI models for us. The cost at this point is quite untenable without understanding the value. So, I believe that achieving a balance between cost and value is another area or a gap that the industry will need to focus on and address pretty quickly.
Responsible AI: How do you think about it as an IT leader? What does it mean to you?
There’s a lot of discussion about this, I’m sure, in most companies. For us, there are a few things that we go off of.
First, anything that we build, we very clearly say, “Do not let this be the only decision point. This is but an input into overall decision making.” So, in a sense, AI can possibly do a task, but a job is a very different thing. A job includes cognitive aspects and we don’t believe we are there yet with AI as an industry. The cognitive aspect isn’t close to where human beings are at.
So, we don’t put AI in places where we really need to make decisions. We look at AI as an augmented decision-maker as opposed to being a decision-maker in itself.
The second reason for concern is that we don’t indiscriminately send data outside the company to external AI models. We are very careful about how we use our data and treat it as an asset.
Finally, we focus a lot on explainability. We try to understand why an AI model, or a Generative AI model, provides the inferences that it does. There is a team that’s starting to look at explainability in AI.
Balaji Viswanath, Managing Director of Emerging Tech, Enterprise Architecture, Software Engineering & RPA at Tyson Foods to our CXO of the Future Podcast (“The Three Questions” edition). He joined Tyson in June 2018 as Senior Director of IT Architecture. Balaji is a Technology and Data Science executive with 25+ years of experience in his field.
He is a technology leader with a passion for applying technology to transform businesses at fundamental levels. He is particularly passionate about bringing in new technologies to solve business problems and build high-performing teams. Balaji has extensive experience working with business groups across verticals to envisage best-in-class digital experiences and translate them into robust Technology solutions.
His core competencies lie in working with business partners to identify large value-add opportunities by applying Data Science, Machine Learning, and other cutting-edge technologies.
He has led several Digital Transformation and Multi-country/Multi-Channel global platform rollouts in E-commerce, Digital Marketing, Web, Sales IT, CRM, and Supply Chain domains.
During his career, he has implemented state-of-the-art technologies such as Adobe Experience Manager, Intershop Enfinity, Drupal, etc. across large-scale enterprise IT. He is also a proven Change Agent, and has led several teams through grassroots organizational changes and transformations including transitions to managed services.
Today we welcome Maria Latushkin, a technology and product executive who has spent over 20 years leading Product, Engineering, and Operations teams transforming technology for global brands including Walmart, Peet’s Coffee, and most recently Albertsons.
As Group Vice President of Technology and Engineering at Albertsons, Maria is responsible for all aspects of technology for retail, payments, and supply Chain, thinking through the future of retail technology and spearheading the digital transformation of the supply chain space.
Prior to her current position, Maria held CTO roles at various consumer technology companies including Omada Health and One Kings Lane, and led technology and product teams at Peet’s Coffee and Walmart.com.
Question 1: First Job: You’ve had a very technical background for a long time. It’s awesome to have a female leader in technology. Could you talk a little bit about your background and how you got to the position where you are now?
I’ve actually had quite a meandering career. But I like to think about it as all of the stars aligning for this specific purpose in life. I’ve been in large companies like Walmart, and really small Series B companies, with most of my focus around some sort of consumer experience.
I’ve also ventured out into healthcare. And now working for Albertsons, I feel like this is all coming together. There are both retail and healthcare aspects in my current role, within wellness and the pharmacy. And it’s really about trying to create better value, improve consumer lives, and develop relationships with the customer through the technology that we leverage.
It’s really exciting to be where we are, where the whole industry is being redefined.
Question 2: Generative AI: Everybody’s talking about it. But you, as a business leader, have seen other cycles before. Could you put a little context around Generative AI? Is this the highest priority for you right now?
I’ve seen these different innovation hype curves, but this one feels different for me. And the reason it feels that way is that it’s not just a new technology, it has the potential to fundamentally transform how we work (as opposed to just doing things faster). And that’s why I find it both exciting intellectually, but also transformative from the business perspective.
It can create or amplify the business models that we have right now and unlock new opportunities and capabilities for us. So it’s something that’s a very high priority.
And, as always, when you have something new, you also want to be careful about how you introduce it into the workplace, and the enterprise. You want to be responsible about it. We have this joke about Generative AI: “With great power comes great responsibility.” It’s a very powerful technology with a very powerful capability that must be governed appropriately. So there’s a lot to think about, and a lot to do. And then it’s: How do you scale it? How do you make it a fundamental piece of your enterprise business?
Maybe you can unpack that for us. I hear a lot of CIOs are doing experiments internally, but at the same time are focused on employee education. Could you talk about some early learnings?
There are a few different areas we need to focus on: first, investment is really hot right now. We’ll need to invest ourselves, and also become better and smarter through partnerships with other companies that have made their own smart investments. When you think about all the copilot capabilities, I do believe this will help enterprises to be much more effective.
And then finally, you need to think about the solutions that you create as a development organization. These will likely be more unique, more bespoke. Something that has specific value at a given company, and every company will be different.
Generative AI is still new and it’s already wonderful at certain things (while still learning others). There are going to be more “Aha” moments and early findings. So it’s key to get started where it’s strong, and not just look at it as a magical unicorn. Gen AI is going to be able to solve certain things, but not others, just yet. But as time goes by, more and more opportunities will become available.
So we have to think about being deliberate and thoughtful around what it’s already good at right now, what’s coming down the pipe, and what might be further out. Then, you try to figure out the phases of engagement.
The other optic here is thinking through quick wins and balancing those with impactful change. It’s not just prompt engineering, right? You also have to figure out the technology, the architecture, the security, the data governance…etc. You have to educate your teams. And so you want to both prove the value and also create a space within the use case to do all those things.
And you want to make sure that you pick the right use cases and the areas that have the highest feasibility. Do you have the data to make it happen? Can you really stand it up and get it off the ground?
And at the same time, you also want to start thinking about the areas that will provide the greatest impact: something that’s meaningful change. That will probably take longer.
Question 3: New metrics: You and I talked a little bit about your way of measuring impact or metrics. I’m curious, as you think about planning for next year, there are lots of use cases where Generative AI could be used. How will you go about deciding where to put attention? I love the idea of quick wins, but ultimately you said this could be quite transformative. So how do you balance all of that?
We try to evaluate two things: One is productivity. Is this something that will help us? Will it amplify what we’re doing as an organization? Will it help us achieve something in an easier and or faster way?
Those initiatives very often fall in the quick wins situations, by the nature of the fact that they give you productivity. It’s something that’s supposed to give you results faster.
And then you always want to pick something more strategic. And yes, it will take longer, and needs to be evaluated against the long-term impact.
You have this opportunity to start building things. I presume you’ve got new use cases. But then we’ve been in an environment for the past decade or so where we’ve been buying solutions versus building. And now we’re in a struggle where you may have to build things that are unique to your use cases. What are some of the technology gaps or issues you need to address? Where would you like more investment? On technology? Or is it in people and processes? And how do you balance the traditional ‘Buy’ versus ‘Build’ equation in this new sector of AI?
It’s interesting, I actually don’t look at it as a struggle. To me, it’s an extension of the same thought process. When you identify something that provides a utility to your organization, it makes sense to see if someone else is doing it and doing it well. In that case, you can buy it, and then you wind up seeing the results and benefitting very quickly.
But if you have things related to your IP, or unique to your knowledge or business model, you may not find something readily available in the market as a generic solution. In that case you’re going to have to build.
It’s the same logic that a lot of companies apply to the regular buy versus build.
In order to build, you will need to acquire more skills. You will need to train your team on this and find ways to be proficient. In some cases, you may be able to shoot for a hybrid solution that extends some off the shelf models that are already close. So maybe you don’t build things from scratch, but it’s also not a SaaS product right away. You’re able to take a platform and extend that platform, making it a little bit more unique, and a better fit for your needs.
Question 4: What are some of the issues or roadblocks, or maybe even gaps that you believe need to be solved before you can fully execute on this? What are the headwinds you’re facing?
I don’t look at them as roadblocks in the sense that we have to solve all of them before we can make progress. To me, they are those forces that sometimes play against you, and other times you just have to keep in mind while making progress towards your goal.
As this is a new discipline, some of these roadblocks will be new and unique, or even use case by use case. So as an example, when I think about the financial aspect of being able to educate people on FinOps, it would make a big difference in certain use cases, but in others may not be necessary at all.
The same applies to data quality and availability: depending on the use case that you’re trying to address, it may be a really big roadblock, or it may not matter at all. That’s part of the way you want to try to evaluate. Do I have all the things that I need to make my use case successful?
And then you need to think about evaluation and validation. How do you know that your use case will produce results you’re willing to stand by? And how are you planning to approach it? This is the whole idea and the whole discipline of data governance. You have to get this right from the very beginning and you have to understand your point of view. What are your guardrails, ethics, and social responsibility? A lot of education needs to happen, and the regulatory side of the house is constantly evolving. But this part is important, new, and ill-defined.
Finally, you must educate your team and upskill them in a variety of ways. It can feel daunting because it’s so new, so figuring out ways to be practical is probably the most important part. People may be excited, but they also may be scared, so you must make sure that you educate, create awareness, train, and figure out how to reach all of the right audiences in the manner that would be important to them.
How do you think about responsible AI as an IT leader? Do you educate your board? How does Responsible AI come together in your mind?
I don’t think it’s one person’s job to figure this out. I do believe it’s important enough that leaders of different disciplines within a company will come together and make it their priority. And so, depending on the structure of the company, it could be different people. But I do feel that this is one aspect that must be addressed early, and people have to be really thoughtful about it in executive leadership.
Maria Latushkin is a technology and product executive specialized in Retail, Payments, and Supply Chain. She is GVP, head of Technology and Engineering at Albertsons, a famous grocery short chain all around California, and the West Coast, where she is responsible for all aspects of technology for Retail, Payments, and Supply Chain, thinking through the future of retail technology and spearheading the digital transformation of supply chain space.
She is a high-energy, innovative technology executive accomplished at aligning technology strategy with business goals and delivering new business capabilities through technology.
Maria has spent over 20 years leading Product, Engineering, and Operations teams transforming technology for global brands including Walmart, Peet’s Coffee, and most recently Albertsons.
Before her current position, Maria held CTO roles at various consumer technology companies in the consumer and healthcare space, including Omada Health and One Kings Lane, and led technology and product teams at Peet’s Coffee and Walmart.com
Last week we hosted a small wine and cheese event here at the Mayfield offices to discuss OpenAI’s slew of recent announcements. The core topic was whether or not this was an opportunity for startups, or a death knell. The general feeling is that startup opportunities are shrinking – but there will still be segments of the market where they have the opportunity to dominate. There was also still a lot of debate around the topic – the hallmark of a still-nascent market.
Today we welcome Ekta Chopra, a Visionary IT Executive and Digital Expert who has invested her entire career in transforming private equity-backed companies with cutting-edge technologies, high-performing teams, and digital technologies.
With over 20+ years of Technology experience from private equity and retail companies, she joined E.L.F. in 2016. Before having her current job as Chief Digital Officer, she worked as Director of Technology of the James Irvine Foundation for three years, where she was recruited to drive a complete transformation of IT team, systems, and operations for this foundation committed to enhancing the quality of life for people in California. Before that, she served as VP of Technology for Charming Charlie, a specialty retailer that grew from 17 to 120 stores nationally.
Ekta holds a B.A. in technology & operations management and a minor in finance from California Polytechnic State University. She is also a member of Silicon Valley CIO/CTO groups as a thought leader.
Question 1: First Job: You’ve been a digital officer for a while, could you talk a little bit about your background and how you got to the position where you are now?
I was born in technology; a tech geek from the beginning. But I had the opportunity to work in many different domains from aerospace to private equity, to women’s fashion accessories to reporting to the chief investment officer for a foundation, to getting my dream job at E.L.F. Beauty, which truly has been like a rocket ship. And that’s what we like to call ourselves: we’re sort of on this rocket ship with a lot of superheroes doing some really amazing things.
So, you know, I moved away and pivoted a bit from information technology to digital transformation in an era where e-commerce was really pivoting from tech to digital. And that’s something that I’ve been able to embrace and really grow at. So that’s, in a nutshell, my journey.
Question 2: Generative AI: You’ve had a long journey working digital strategy, could you put a little context around Generative AI? How does all this fit together? It isn’t necessarily a brand-new thing, but an evolution, right?
Absolutely. I’ve been doing what we call power-up sessions at E.L.F. to educate the whole company. And I’d say 1956 is when generative AI was born, essentially. It has had many evolutions over time. So, AI has been there for a long time. ChatGPT just made it very accessible in 2020. And today it almost seems like, you know, every conference, every boardroom, every investor is thinking about it. This is an evolution. Not a revolution, but an evolution.
Now is the time to find a way to really embrace it, because it is something that I see has a lot of potential from a consumer lens, which, as you know, is very dear to my heart. But then, as a public company, I also must think about it from an employee efficiency and employee experience perspective. I think it has tremendous potential.
It sounds like it’s becoming a higher priority for you and your business. Where does it fit in the prioritization you mentioned? You’re getting looked at by Wall Street, I’m sure your board is asking for more things. How high priority is it?
It’s really high priority for us. But I would say that we have already been doing a flavor of it. So, if you think about our digital business, it’s doing extremely well year over year. E.L.F. has had 18 consecutive growth quarters. We’re now the number one favorite Gen Z brand, and you don’t become the number one favorite Gen Z brand unless you really understand your consumer. So that comes with offering personalized experiences. But AI will allow me to offer hyper-personalization, which I’m really excited about when it comes to E.L.F.’s products.
And if I think about that and put it in perspective, the amount of creative, the brand messaging, and the content that you need, is exponential. How do you do that? You know, it’s not humanly possible without hiring a bunch of folks. So how do you do that effectively with either co-pilots or for your everyday content? You need a content supply chain that’s driven by AI.
I think that’s where I feel a huge opportunity, from a productivity perspective and really engaging our consumers. So that’s been our focus area as I think about our journey and acceleration.
Extra question: Could you talk about some early learnings, or what you have experienced in the effort of having more data and getting closer to your customer and understanding their needs?
I think the early learnings really are: ‘You have to be grounded in reality.’ There is, of course, all the excitement and the innovation that’s been possible since 2020, but I think there’s also the reality of us being a public company.
So, if I think about our focus areas and how the learnings have shaped them, it’s leveraging this concept of prompt engineering. And it’s also about education: getting everyone in the company comfortable with co-piloting using AI. It’s really important, right? If they don’t know how to ask the right questions or prompts, they’re not going to get the right results. And you’re not going to be able to train the AI the way you want to on your brand, voice, tone, or your own persona as an employee. So that’s number one: educating and getting our employees trained on it.
Second, whether it be Microsoft, Slack, or Salesforce, everyone is coming out with co-pilots. So, we need to test and learn. We can work with existing vendors to be the guinea pig in some cases, because as long as they’re trusted vendors and we’re being mindful about our use cases, it’s a good use of our time.
The third piece is that we have to consider where it makes sense to plug in some of platforms that are already built for certain use cases versus building new things ourselves. For example, why do we need data analysts? Why couldn’t we build something to provide access to our data at the fingertips of different people? This would have the potential to create a lot more speed an actionability.
Furthermore, there’s not just one model. You have Llama, you have Bison, you have Open AI. Each one needs to be trained differently, so they won’t necessarily train the same answers, or react to the same use cases in the same way. So how do you play around in that sandbox?
And finally, AI governance and security enhancements are critical. You can’t afford to not be thinking about them, especially as an enterprise leader. So, we need to stay grounded in those realities. There are things where we’ll go deep and things where we’ll just tiptoe a little bit and just test and learn.
So, is that a planning exercise? Or is it really just better communication throughout the entire project?
Great question. It’s a combination of both. You start with the planning, and then you take it all the way through execution. And you build the muscle memory so that it becomes almost second nature that you must communicate. So, it’s a planning thing as well as a communication thing.
Question 3: New metrics: How will you measure success? You’ve got both internal productivity opportunities and then you’ve got consumer brand, and of course, customer adoption. So, what are the unique metrics that generative AI is creating for you?
I think it falls into three buckets.
One is: “What impact is it having on our business?” And that could range from increased revenue, new revenue streams, reducing cost of how you serve, or your consumer, or things like improved consumer experience through the implementation of chatbots. The impact on your business is very real and quantifiable.
Then there is technical feasibility. Is it really technically feasible? Is it costing you too much? You know, as you try to build these cases, you have to also take that into consideration.
And I think the third is availability of data. You have necessary data models that you’ve trained to get solutions that are actually making an impact. So, you have to be able to measure it. You know, we have many use cases right now that we’re trying out, and some of them frankly don’t even make sense. They’re not even AI use cases. I think it’s about evaluating where the juice is worth the squeeze.
Bonus: What are some of the technology gaps or issues you need to address when thinking about all this from a venture capital perspective? Where would you like more investment? Is it more about technology or are people and process more of the gaps for you?
I would say it’s not one thing you know? My Head Developer or Engineer would say there’s always a caveat. So, in this case, there’s a great degree of complexity. There are technical challenges, of course, which are huge. How can you really make this technology fit into your ecosystem and work with many of the other platforms?
Then, there are data challenges. You’re working with a huge amount of data. How do you build something that is going to move at the speed of the business? We work at E.L.F. speed. So, for me, it’s really important that whatever I bring in can actually move quickly.
And then there are ethical challenges. As a brand we’re extremely grounded in our purpose. E.L.F. is for every eye, lip, face, and skin concern. I cannot discriminate. So, how do I make sure that the models I’m building are ethically right, that we’re serving the consumer the right color shade, matching, and so forth? And we really want to make sure that we’re very sensitive to that.
And of course, the nice bow on top is security and privacy. That’s always a concern as a public company. I would never even consider prototyping or doing something that already inherently might have security concerns around it.
And then of course, an issue I know my employees are concerned about is how this is brought into the organization. Are you doing this to get rid of jobs? And the answer should be no. It’s to make sure your smartest people are as effective as possible, and you’re actually making their jobs easier. So, the framing of that is so important as well.
Question 4: What’s a generative AI takeaway. If you had to tell Grandma what Generative AI is, how would you go about describing it?
I would say it’s like a Magic 8 Ball because you don’t know what’s happening on the inside. But it’s a more effective Magic 8 Ball that is capable of providing you precise information, depending on what you’re asking. And the more you engage with it, the more fine-tuned it is to what you’re looking for. It’s going to improve the broader quality of the work individuals are doing inside the business, and can serve in many new revenue streams, while creating new ways to engage with consumers.
Ekta Chopra is a Visionary IT Executive and Digital Expert who has invested her entire career in transforming private equity-backed companies with cutting-edge technologies, high-performing teams, and digital technologies.
Digital Expert, Metaverse and Web 3.0 enthusiast, Board Advisor with experience in private equity and retail, she has over 20+ years of Technology experience. She has invested her entire career in transforming private equity-backed companies with cutting-edge technologies, high-performing teams, and digital technologies. She also leverages cutting-edge technical solutions to maximize operational efficiency and drive unprecedented results.
She currently works at E.L.F. Beauty as Chief Digital Officer. Ekta holds a B.A. in technology & operations management and a minor in finance from California Polytechnic State University. She is also a member of Silicon Valley CIO/CTO groups as a thought leader.
We recently hosted our latest CXO Insight Call: “CXOs and Gen AI – An Investor Perspective on the Technology Breakdown,” morning of Tuesday, October 31st, where we hosted Vijay Reddy, Partner here at Mayfield, and Isaac Sacolick, Founder and President at StarCIO. In our content below they speak from an investor and practitioner’s perspective on the tremendous opportunity and ongoing adoption of generative AI, which is driving a new AI-first enterprise IT stack.
IT leaders across all industries are already thinking about what their top of mind priorities will look like, including how they plan to educate and experiment, manage their blue sky planning, update data governance policies, and so much more. Isaac and Vijay discuss what they’re hearing from hundreds of tech executives in the market, and cover many urgent questions around LLM adoption and strategy.
Vijay has been a fantastic addition to the Mayfield team, joining us from Intel Capital earlier this year as our new AI-focused partner. His investor journey began in 2014, which just so happened to be the same year that deep learning began smashing through some fundamental benchmarks. This marked the early innings of the deep learning era. During that time, trillion dollar markets opened up across a variety of different industries. AV, medical, satellite imagery, and so much more greatly benefited from these disruptions. It was a ten year investment period for many AI-focused firms.
A recent survey in McKinsey suggested that the technological advances we’re seeing in AI today are ten years too soon for a variety of different use cases. This includes rote tasks such as math or coding, but also cognitive activities that are further afield, like dialogue, video generation, and a higher order of understanding and interpreting natural language. We’ve reached the point where we’re going from repetitive tasks to cognitive tasks – this is a big moment, especially when looking at very large markets.
AI won’t necessarily replace humans, but humans utilizing AI may be substantially more productive than humans who aren’t. And because of the human aspect of all of this – the markets that AI will be replacing have to be considered differently. People tend to look at markets and say: “What is the TAM for accounting software?” But in the case of AI, they’ll have to say “What is the TAM for accountants?” and start working back from that. That’s around $20B, when the software TAM is only a few hundred million. So startups are primarily approaching markets that have huge human capital, and are trying to greatly augment humans within those markets.
In the last cycle, as mentioned prior, AI started with the data scientists and eventually trickled down to the business users, but now we’re seeing the reverse. Business users are eagerly coming up with use cases that are then passed down the pipe to IT, data analysts, and data scientists – with the implication that they will come up with a solution. Because business users are the originators however, there is an order of magnitude more people touching AI in their daily lives, and who are willing to go to bat for it on budget.
Another big difference is that in the past companies had to build enterprise applications very tailored towards one persona. If you were a product company (as opposed to a sales company) the data sources would be entirely different. But now, some of these new gen AI companies are cutting across different use cases, budgets, personas, etc.
Finally, as models continue to scale up, emergent behavior may continue to unlock new use cases.
We’ll see a lot of capital go towards enterprise infrastructure, and even though the number of deals has slightly decreased, the funding has very much increased. What that indicates is that a few companies, particularly the model building companies, have raised a lot of capital. This makes sense: they’re competing with the giants – they need better algorithms, compute, data, etc.
This is roughly where the capital is being allocated today, but it will of course continue to change over the next 5-10 years. We’re seeing a lot of copilots, a lot of AI assistants, and a lot of focus on marketing and sales. Right now we’re at the peak of the hype cycle, but that will change over time. Startups today are out there building as many different hammers as they can, and then going out to look for nails. Practitioners must help identify where those nails really are.
On the fundraising side, we’re seeing a bimodal distribution. Some startups with just a couple million dollars can use gen AI to get to an MVP really quickly because they’re using open source tools or publicly available datasets. On the flipside, if you created your own model you have to raise a lot more, like a $20-$40M seed raise before you can even start selling anything. So the bifurcation is really between companies who are building models vs. those just taking things to market directly. Text is very oversubscribed right now, but videos, images and music are all wide open.
Pressure from the top means that organizations are going to have to come up with their AI strategies in a bit of a hurry. And they’re not wrong, generative AI is likely to make a significant impact in IT. The same way that mobile devices in the past led to the consumerization of IT, generative AI is leading us in the direction of the consumerization of search. Unstructured content and documents can now be used to make customers and employees better informed and more intelligent. IT should already be leveraging some of these capabilities today, including things like:
CXOs should start by getting a ground floor view of what people are experimenting with, or what people want to experiment with. IT and marketing are two fruitful areas with a lot of tools and built-in platform capabilities that you can take advantage of.
The second step is building out guidance with IT leaders within the organization, especially where there is risk around sharing IP. Take a look at what people are doing with the tools you already have. All the big players like Atlassian Intelligence, GitHub, Adobe, etc. are starting to include point solutions for generative AI that give you a narrow, siloed view of your enterprise data. And now you can use it for things like answering a service desk call, or processing information, or doing a search within your Gira tickets. There are a lot of quick and easy things to do.
Finally, from a developer’s perspective, it’s time to start thinking about how to make your own data accessible to LLMs before you even consider building your own. And as you dive into that technology you’ll start to see a lot about vector databases, embeddings, etc. and that will take your information sources, compact them down to a vector type of information (whether you do that yourself or with tools as the tools mature), and enable you to plug your data into either private, industry, or open LLMs so you can start making really intelligent decisions around that data. Things to bear in mind:
As CXOs start going up the stack and thinking about AI strategy and how it should be presented, there are a few things to consider:
Data governance
Guardrails
Communications
First of all, this is something that needs to be considered on a timeline of 2-4 years down the road. Places like healthcare, education, etc. that have large untapped corpuses of IP (that have probably remained untapped over the years because the focus has been on relational data and analytics) can now be prompted against.
CIOs will need to project where their specific IP is going to offer advantages and how that will play out across their industry. This is the first step in defining a game-changing LLM strategy. The second piece of that will of course be cleaning and prepping data for those private LLMs.
Finally, there’s a notion that AI is going to take over for humans and make everyone’s decisions for them, but this really comes down to figuring out where decision-making authority really lies. Machine learning and LLMs are only aids in helping people make smarter and faster decisions.
The board will be asking a few different questions that CIOs should bear in mind:
IP, data and content behind the firewall
Experimental culture, personalized experiences and workflow
Governance: who and how
Every software company is in a race to hook up their apps to Open AI, but you have software for CRM, ERP, HRS, and all kinds of other use cases. Is there a world where there will be specific LLMs we plug our business processes into? Not just Open AI, but very specific LLMs?
Eventually every endpoint will have something like an agent, whether that’s a CRM or otherwise, and a lot of the open source community is currently in the process of building these agents. And we believe that at some point these agents will start communicating with each other. We don’t have the frameworks yet for that, but someday your sales agent may start communicating with your marketing agent.
What is the role of data going to look like going forward?
Large enterprises are now well aware that data is the moat, and most startups have now recognized that enterprise data is where they want to play. So, there’s definitely a lot of tension today between incumbents who are trying to get insight into their own data (and monetize it) and startups, who are getting really smart about how to get at that data (even combining the data from many different companies – creating positive network effects). One question will be whether or not enterprises team up to create industry-specific LLMs themselves. Technology isn’t what will hold things back – it will be trust.
With the value of data now clearly understood by all, could there be a mid-market kind of play for matching and consolidation and aggregation of data?
If a startup wants to have a competitive moat, but you can scrape the web using an open-source model, the moat for that company won’t be in the technology or in the data. So, we encourage startups to think about how they can get access to private pockets of data. For example, looking at healthcare and genomics, there are a number of companies trying to aggregate some of these data sources. Unfortunately, this requires a lot of work with the other players across the ecosystem in order to successfully make things happen. This could be legal/privacy-related, or it could be that company business models themselves are prohibitive.
What are the primary use cases where enterprises can get started? Seeing some early innings in SecOps, DevOps, Code Generation, Data Analytics, etc.? But in classic IT functions, where should we be looking for time to value?
These are very similar use cases to what we are seeing. From a non-IT standpoint, we’re also seeing a lot in the back office. Front office will be slower because there’s the issue of having a human in the loop, but in the back office we’re starting to see things like software developers becoming more proficient with tools for code development and code completion, as well as code search. There are also a lot of different companies looking at different data sources across IT, and extracting insights from that. Five years out we’re going to see more agents being used.
We’ve deployed Claude to our entire company and everyone is using it for summary and query-based use. We don’t need to go much further than that because our other primary business applications are releasing AI functionality over the next 6 months. In light of that, do we need to invest in generative AI? Where is this profit coming from that’s expected to dominate the market? A lot of this seems off-the-shelf or free?
One way to think about this is that a lot of the costs today are still being figured out, and companies large and small will want to know if customers are really willing to pay for these extra features (or not). Today things are still being subsidized and companies are still in the ideation phase, but eventually everything is going to settle out and it may not be so inexpensive to ride along with the majors.
How do you communicate around concerns the workforce might have regarding how this could impact them or their role?
We’ve had this struggle every time there has been a technology that moves up the stack and challenges the types of things that employees are doing. Whether that’s robotic process automation or low code technologies, there is always wariness around this method of moving up the stack. The CIO formula around all this is fundamentally simple to say and hard to execute. It’s really about bringing people together, making reasonable projections over what people will be doing with these technologies over the next 6-18 months, and then really thinking through what people’s jobs are going to look like when that surfaces, and how you will really need to wind up training and preparing people to do things differently.
With the rapidly changing pace of technology, employees will need to learn new skills, but which skills, and how quickly? There is a plethora of learning all around. Furthermore, the standard way of learning (going to school) is somewhat ineffective in the current circumstances. So how are universities adapting to this kind of change? And how is AI actually being used to create new kinds of personalized learning?
In terms of employees and learning, this can seem chaotic, and in some ways you want a little bit of chaos in terms of giving people the freedom to ask the right questions. But putting down some governance and figuring out what tools and what data people should have access to is important as well. You want to open up the floor to your employees to ask a lot of questions, and then narrow things down and say let’s apply our learning activities in these initial channel areas, let’s provide some tooling around how you can collaborate and how you can share your learnings, and most importantly put some structure around the data and tools that people are using. Start with a wide funnel and then narrow things down around how people should spend their time.
Education in general is going to run up against a lot of disruptive factors, it should have already over the last few years, the pandemic was the spark in terms of how people were learning virtually. And now, when you think about the education of the future, it must include prompting, and the creative exercises necessary to help educate people in their future roles. We went from digital natives, to now, AI natives coming into the workforce over the next ten years. Higher ed in general will ideally want to take advantage of this new technology, instead of persisting in a linear learning model.
What is the balance between moving fast and moving securely? How will these regulations be consumed on the startup side and at the enterprise level?
The one thing we have to learn from the past is that saying no and locking out employees from doing anything in the space is a recipe for disaster. The hard thing this time around is going to be getting ahead of the board (and those same employees) and saying “Look, here’s the playground, problems, and who and how to collaborate.” Finding some good fruitful areas and saying “Let’s focus here first,” is a good start. There’s a lot to be thinking about like the lack of explainability, and the lack of attribution that these LLMs are doing today. When we do machine learning and analytics we know how to create synthetic datasets, do automated testing around it, and start seeing how the boundaries work. There’s still a lot of technology and know-how and skill to be able to do that, but those tools don’t even exist for LLMs yet. So if we create an LLM with whatever level of expertise it has, where do its boundaries begin and end in terms of what questions it can legitimately answer? We don’t have a good answer for this today. So that needs to be top of mind when talking about LLM Ops. Is this particular LLM with this data ready for production use? Or what level of production use is it ready for? I don’t know if Google and Open AI’s open approach of seeing what happens and cutting back if they run into issues really works well in the enterprise. So you have to take things slow and think about the data governance side of it, while making your IP ready for vectorization, and for embedding, once LLMs get to where they need to go and there’s good technology out there to do validation.
What are your thoughts on what came out of the White House yesterday?
From an investment standpoint this is very exciting news. We’ve been looking in data ops quite heavily, including PII data and access control. From the model standpoint, we’re thinking about things like: How do you score a model? How do you red team/blue team a model? How do you add guardrails to a model? How do you reduce hallucinations in a model? These are all very exciting investment opportunities for us and our goal is to definitely help address some of these issues. But, we think it’s an evolving space where regulations will change. Eventually enterprises could need insurance, or protections, or who knows. But these are going to be very dynamic and interesting challenges that both enterprises and startups alike will need to react to.
The good news here is that the government is getting involved. Yesterday was a lightweight first step in creating a directory and technology companies should be open to doing that. As the government learns more, in the back of their heads, they want to make sure that the people of the country are safe and that our intellectual property is safe. But just like on the enterprise side: We can’t sit back and wait. Our adversaries will be implementing these same technologies with far fewer regulations and scruples around them. So, we need to keep the innovation going, but start learning what the government’s role should look like as capabilities continue to expand.
We recently hosted a call with Karthik Bharathy, Director of AI/ML services at AWS, who spoke on recent strategic announcements and AWS capabilities – specifically on how AWS’ AI/ML offerings look, how you can leverage them, and how to best work with the AWS team as a startup.
At AWS, the primary goal today is figuring out how the business can take advantage of generative AI, beyond just simple text and chat use cases. What led to this moment was the massive proliferation of data and compute: it became available at low cost and a very large scale. So, machine learning has experienced a ton of innovation over the 2-3 years, which has accelerated the prior efforts tremendously.
Generative AI is a fundamental paradigm shift from the AI of the past – you’re trying to generate new content, powered by foundation models, which are pre-trained ML models leveraging vast amounts of data. What’s important is that these foundation models can be customized for specific uses cases, giving them more power and relevance
From an AWS standpoint, ML innovation is in their DNA – going all the way back to e-commerce recommendations, or picking the routes where packages can be stored, Alexa, Prime Air, or even Amazon Go where you have a physical retail experience. These products already incorporate machine learning and are backed by foundation models
Today, there are over 100K customers across different geographies and verticals, all using AWS for machine learning, and all of these customers are already in production.
There are four key considerations for startups when spinning up ML capabilities:
Amazon Bedrock is a fully managed service that offers high performing foundation models from companies like AI21 Labs, Cohere, Anthropic, Stability AI, etc. including foundation models coming from Amazon (Titan models).
This is a serverless offering, meaning you don’t have to manage any infrastructure when you access these models – all you have to do is use APIs to interact with them. You can also customize these models with your own data (e.g. fine-tuning or RAG)
You have the choice of taking advantage of an on-demand mode where you look at the input and output tokens, and then essentially index on what your pricing will be, so that you can project based on your current application needs (vs. future application needs). Whether this is coming from NVIDIA, AWS Inferentia, etc. – it’s all under the hood – you’re not exposed to instances. There are even capabilities like instance recommender that can suggest what’s the best instance for a given model – it really just comes down to the use case
There are many different models today, and this list will continue to expand. You can try them all in a sandbox environment via the AWS console.
The Bedrock service is HIPPA compliant so you can use the models in GA along with Bedrock for your production use cases
Getting started with Bedrock:
In terms of pricing there are three different dimensions:
If you’re an ML practitioner who wants to try out an open-source model, and have access to the instances, you can use Sagemaker JumpStart. This is a model hub where you can access HuggingFace models (or other models) directly within Sagemarker and deploy them to an inference endpoint. Fine-tuning is different from the Bedrock experience – you work via a notebook where you make changes to the model in a more hands-on way, so if you’re an ML practitioner who is very familiar with the different techniques of fine-tuning, it gives you a lot of knobs and flexibility on how you can build, train, and deploy models on Sagemarker
Foundation models, however, are just one piece of the puzzle. From a process perspective, there’s a lot more to the orchestration piece. Users typically want a task to be accomplished (vs. just interacting with data), so if you have an application, but you just want to book your vacation, that’s going to involve a series of steps (e.g. understanding the different prices, selecting the different options, etc.). So, it’s a process in and of itself and that involves more than just interaction with the model – you must also interact with the data, and a bunch of APIs on the back-end, and so on. And at the same time, you want to ensure that security is tight, because while there’s orchestration, you also want to meet your enterprise cloud policies. This can take a number of weeks if you do this on your own, and Amazon Bedrock has just announced “Agents” to make this a lot simpler
How do Amazon Bedrock Agents work to enable generative AI applications to complete tasks in just a few clicks?
Differentiating with your data is key – It’s pretty evident, but while foundation models can do a lot of things out of the box, their impact is vastly amplified once they are fine-tuned with your data sources. So, net data is your differentiator. You’ll get higher accuracy for the specific tasks that you’re after. All you need to do is point to the repository of your custom data, then point that to the foundation models. The foundation models will do the training run and produce a fine-tuned version of the model that you can use in your application
The customer data you provide to fine-tune the model is only used for your own, newly made, fine-tuned model. It won’t ever be used to improve the underlying model that Amazon is providing. AWS can’t access your data and don’t intend to use it for improving their own service. Everything is being generated in your VPC, so there are enough guardrails in place on who can access the data or the model. In fact, whenever a model is being used, e.g. a proprietary model, the model weights are protected so that consumers of the model don’t get access to the model. At the same time, the data that’s being used to fine-tune the model is not available to AWS or the model provider
It’s important to have a comprehensive data strategy that augments your gen AI applications. You have a variety of different data sources and in the case of structured or vector data, in some cases you may want to label the data. There are services in AWS which can be used for labeling the data which will give you more accurate results when you fine-tune your model. Then of course, you also may need to integrate multiple datasets. There are capabilities for ETL, so you can connect all your different data sources. Data and ML governance are also available as you build out your application
When you’re trying to run foundation models, it’s important that you have the more performant infrastructure at the lowest cost. AWS silicon supports many of the foundation models – you have the choice of using the GPUs when it comes to hosting and training foundation models like the A100s and the H100s, there is also custom silicon, AWS Inferentia 2, and Tranium available
Another fantastic way to leverage Amazon, is via targeted applications that enhance productivity. These are a few popular options:
Amazon has heavily leveraged Coursera to provide a comprehensive suite of offerings on the topic.
You can choose the service that you want to learn about + your role in your organization, and it gives you a bunch of resources to learn more. And should you have specific asks on a Bedrock Workshop or you want to learn about Sagemaker Jumpstart, AWS has hands-on workshops where their specialists will engage with you and help set things up. AWS is also investing a large amount of money into their generative AI innovation center, which will connect you with ML and AI experts, so that if you have an idea, they can help you transform that into a generative AI solution
Karthik is an product and engineering leader with experience in product management, product strategy, execution and launch. He manages a team of product managers, TPMs, UX designers, Business Development Managers, Solution Specialists and engineers on Amazon SageMaker. He has incubated and grown several businesses including Amazon Neptune at AWS, PowerApps, Windows Azure BizTalk Services, SQL Server at Microsoft and shipped across all layers of the technology stack – graphs, relational databases, middleware, and low-code/no-code app development.
Karthik holds a masters degree in business and an undergraduate in computer engineering.