We recently hosted a conversation with over a hundred CXOs from our network as part of our ongoing efforts to bridge the gap between the promise and reality of GenAI. IT leaders across all industries are already thinking about what their top of mind priorities will look like, including how they plan to educate and experiment, manage their blue sky planning, update data governance policies, and so much more.
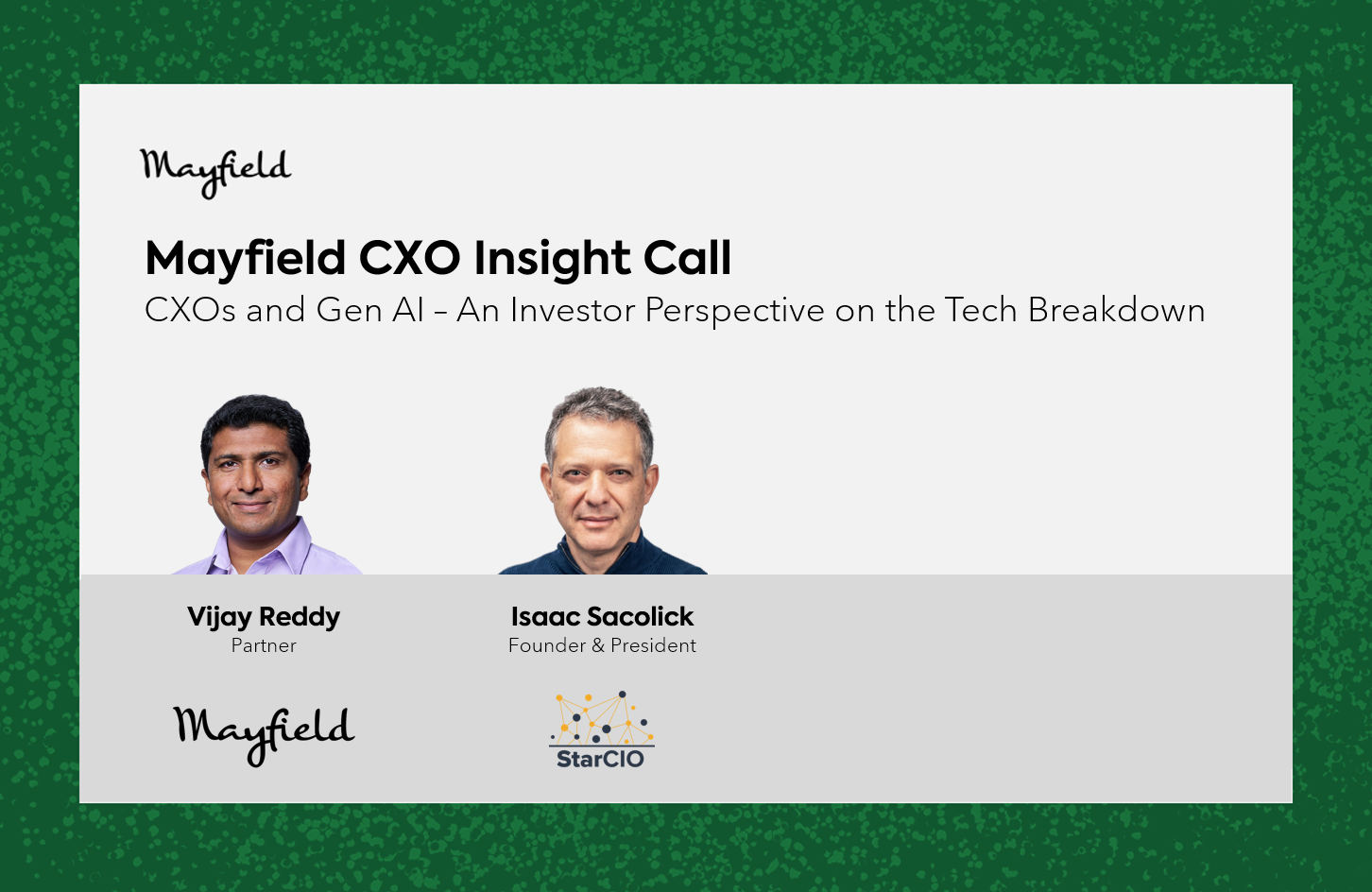
Key Takeaways
- A recent survey in McKinsey suggested that the technological advances we’re seeing in AI today are ten years too soon for a variety of different use cases. This includes rote tasks such as math or coding, but also cognitive activities that are further afield, like dialogue, video generation, and a higher order of understanding and interpreting natural language.
- AI won’t necessarily replace humans, but humans utilizing AI may be substantially more productive than humans who aren’t. And because of the human aspect of all of this – the markets that AI will be replacing have to be considered differently. People tend to look at markets and say: “What is the TAM for accounting software?” But in the case of AI, they’ll have to say “What is the TAM for accountants?”
- We’re seeing a bimodal distribution of funding. Some startups with just a couple million dollars can use gen AI to get to an MVP really quickly because they’re using open source tools or publicly available datasets. On the flipside, if you created your own model you have to raise a lot more, like a $20-$40M seed raise before you can even start selling anything. So the bifurcation is really between companies who are building models vs. those just taking things to market directly.
- The same way that mobile devices in the past led to the consumerization of IT, generative AI is leading us in the direction of the consumerization of search. Unstructured content and documents can now be used to make customers and employees better informed and more intelligent.
- CIOs will need to project where their specific IP is going to offer advantages and how that will play out across their industry. This is the first step in defining a game-changing LLM strategy. The second piece of that will of course be cleaning and prepping data for those private LLMs.
- The abstraction of the stack results in selling to different personas – Back in 2014, the original AI stack was quickly abstracted away. You needed to be an AI expert and know algorithms and also hardware. But then, CUDA came along and you could write algorithms on top of that. And with PyTorch and TensorFlow there came another layer of abstraction. Then finally, with MLOps and SageMaker, eventually you could go directly to apps to write things. So you went from selling to the data scientist, to analysts and business users. And from a core engineering perspective it was interesting to see how different tools developed for different personas.
- There has been an evolution from AI- Centrism to Data-Centrism – Many companies started out as AI-centric companies, but after applying models in practice, quickly realized that those models weren’t fitting their use cases properly (leading to many companies becoming more data-centric). This was a difficult pivot for them, but that transition did need to happen. Data-centrism is more about how to fit the right models to the data. What kind of model needs to be used? How is the data being collected?
Key CXO Considerations for CXOs:
Data governance
- Where is your IP going? Where can it be used?
- Make sure to review SaaS T&S
- How are you accelerating data quality?
Guardrails
- What are your employee use cases and platforms? What tools are being used in what ways?
- How are you validating results? Make sure everyone is helping expose what problems and opportunities are worth solving for
- How are you addressing safety and regulatory?
Communications
- To your board and leadership: What is your clear and articulate AI strategy that’s updated on a quarterly basis?
- To your customers and partners: What are you experimenting with as a company today? What’s your roadmap for tomorrow?
- To Employees: Where are there learning opportunities they can take advantage of?
Stay tuned for upcoming conversations as we all navigate this exciting and changing landscape together.